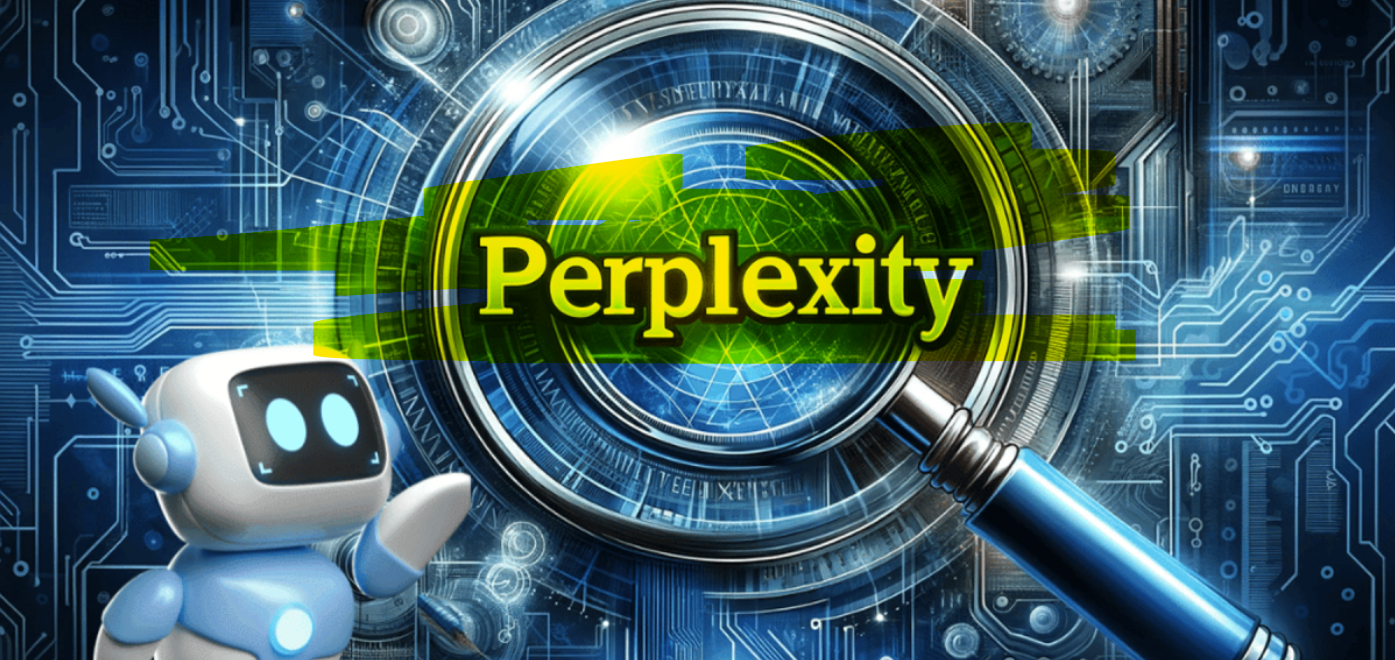
Artificial Intelligence has evolved over the past few years and particularly in natural language processing & understanding. One of the coolest uses for this is to help search engines and information retrieval Perplexity AI leads the way in utilizing large language models for smarter, context-aware search results. Read on to learn how Perplexity BERT is changing Search using AI.
The Power of Large Language Models
Perplexity is based on large language models (LLMs) such as GPT-3 and its successors. Because these models are trained on so much text data from across the internet, they have a ton of information about language and context in them.
LLM can understand the meaning and purpose of a query rather than simply sifting through keywords as traditional keyword-based search engines do. They have the ability to answer complex queries, decode context and implications, and even make sense of ambiguous or incorrect search phrases. Natural language understanding delivers human-like interaction with search engines.
Contextual Understanding and Relevance
Understanding Context – One of the most striking advantages of using Large Language Models for search is their contextual understanding When a user types in an API query, Perplexity’s AI does not merely match keywords; it understands the full context of what leads up to that question and probable intentions are.
For instance, let’s say you search for “apple nutrition.” Many classical search engines could deliver a random smorgasbord of outcomes involving Apple the fruit and Apple the tech giant. The AI of Perplexity is wise enough and it knew from the term nutrition substring that this will most likely represent a fruit. It can prioritize and curate results based on this.
This context enables Perplexity to serve much more relevant results that adhere more closely to the intention of each user. This is cut to the chase rerun, clarification build ask what they are asking.
Synthesizing Information from Multiple Sources
Understanding that Perplexity uses LLM-based search, it introduces another strong skill where you can merge data from multiple sources. Instead of returning a list of links, the AI can read pages from multiple sites & pull out the most relevant information to provide you summarized account.
In such cases, it is difficult to have a one-clear answer – hence aggregations. Bots can aggregate information from various sources most of which are credible/ weigh pros and cons or multiple perspectives save a summary to show the user. This is a big time saver and gives the users an accurate picture compared to visiting ten sources or seeing all data in bits – pieces and stringing them together
Handling Natural Language Queries
Using LLMs allows Perplexity users to search in a conversational format. This allows users to interact with a computer as if they were talking to another person instead of thinking in terms of keywords. Additionally, the AI needs to be able to deal with complex multi-part question and potentially do back-and-forth dialogue to understand that user query.
For example, a user could ask “What were the main causes of World War I that led to World War II? The AI chops this up, recognizes the relation between both halves of the question and delivers a sentence (or three) that links these two conflicts together.
Such a natural language interface makes search more intuitive and user-friendly, particularly for those users who have some trouble constructing effective keyword searches.
Continual Learning and Growth
Probably one of the most interesting things about Perplexity is that it continues to learn and get better. The AI becomes more intelligent in the way it works when users are interacting with i. e., asking questions, giving feedback…
In other words, the system can learn to recognize these types of user intents far better than before and will synthesize information at a higher level accruing knowledge over time about what it knows or does not know. Your search experience is proving better and more personalized searches over time in a virtuous loop.
Challenges and Considerations
The use of LLMs in search offers many advantages but also poses significant challenges that Perplexity has to overcome cautiously. These include:
Factual: LLMs can be gamed to produce plausible but untrue facts Perplexity must have strong fact-checking mechanisms.
Transparency: Users need to know when they are reading content generated by AI as opposed to documented quotes.
Bias mitigation: LLMs tend to learn the bias from their training data. Perplexity works to uncover and mitigate these biases.
Privacy: As the AI becomes more personalized, it is important to ensure user data and search history are protected.
What the Next Era of AI-Powered Search Looks Like
The way Perplexity goes about this is probably the bleeding edge of search tech right now, but I bet it’s just getting started. The further models develop, the more nuanced and informative search will be.
Other details you can expect in future iterations:
File search integrating images, audio, and videos
Advance personification from the user knowledge and personal interest
Syncing with your own AI assistants to search for information without interruptions
Conclusion
The use of Large language models by companies like Perplexity AI is redefining search, making it smarter and more akin to a human experience for users. Using the power of AI to know context understand stuff and talk naturally, Perplexity is setting a new standard for accessing knowledge. As this technology advances, it could be a transformative tool for helping us to learn more quickly, find information faster, and make better decisions in today’s world of Big Data.