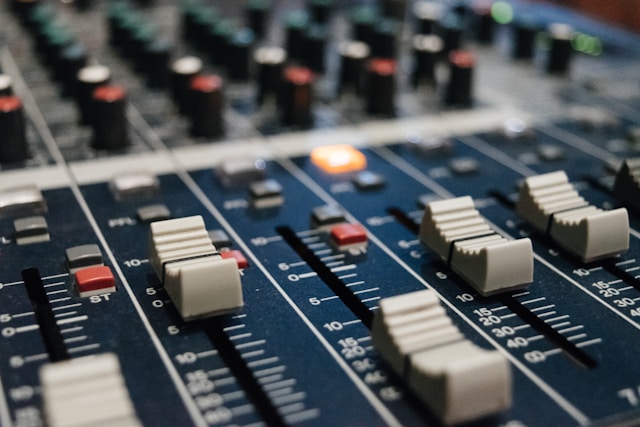
As the landscape for artificial intelligence develops rapidly, Suno AI is a revolutionary technology in music composition. It has gained the interest of musicians, technologists, and ambassadors for AI in general which could change the way we produce or experience music as a whole. But how does Suno AI do this, and what is the science behind music that can sound legitimately human-generated?
I’m going to take you on a journey into the wonderful intricate world of AI-based music composition using partnerships we’ve made and explain how this technology enables Suno AI.
The Fundamentals of Machine Learning and Neural Networks
Sunolabs is based on machine learning (deep neural networks,) or AI. Intended to handle larger datasets and discover patterns from them, they are a set of complex systems based on the human brain.
Deep Learning in Music
Most state-of-the-art music generation deep learning models are based on either Recurrent Neural Networks (RNNs) or Transformers. Models like the ones we trained are well suited to process sequential data types such as music, this is along with being o for generating them.
RNNs & LSTMs: The Recurrent Neural Networks, specifically the more advanced versions known as Long Short-Term Memory (LSTM) networks can keep track of information over time. This makes them well-suited to understanding the temporal details of music, e.g. rhythm and melody progression (Simon 1972),
Transformer Models: Part of the current-gen, transformer models have performed extremely well in the last couple years across AI tasks and music generation is no different. Attention-based models for placing weights on different parts of the input data have been used to help music transformers learn long-range dependencies so that they know what occurred in bar 3 when deciding how to place its output.
Training Data: The Fuel for AI Composition
The performance of Suno AI is highly dependent on the quality and quantity of training data. This data probably contains a huge set of songs, I am guessing…
- Files w/Note Information – MIDI
- Sound Examples (for listening to timbres and textures)
- Music theory that you can grab onto
These data sets determine what the AI is trained on and how, identifying patterns it sees in music to create a mental model that can be used for future compositions.
The Composition Process
Suno AI does not yield what it has memorized when scoring music. Rather, it employs the knowledge of music stored in its memory to compose new pieces. Simplified Breakdown of Process
Seeding: A system is initialized with a seed (which could be as simple as nutrients, chords, or genre)
Model is generated: The model for what note or chord etc will come next in the musical piece based on this seed
Reiteration – the process is looped, running itself anew on each new prediction until an entire composition has been generated.
Processing: More sophisticated systems could include steps of processing the piece to harmonize it and make sure all its elements are musical.
Advanced Techniques in Suno AI
You have the basic principles of neural networks – which would be used as architecture or blueprint,for sure but Suno AI likely uses a number of advanced techniques to improve its compositions.
1. Multi-modal Learning
Suno AI may be trained on not just one kind of data, but a blend from different sources – such as audio samples or MIDI files (files that contain all the information needed to play instrumental music), lyrics and even visual representations of music. This multi-modal approachsters a more complete understanding of music.
2. Style Transfer
Much like AI can transfer the style of one image to another, Suno AI might be able to take the style in a piece of music and apply it elsewhere with combinations we’ve never heard before.
3. Controllable Generation
Such AI music systems typically also feature some control over the generation, where users specify certain parameters (e.g. mood, tempo, or instrumentation) to which elements of the input and output would adhere to a greater extent than others.
4. Reinforcement Learning
Reinforcement Learning methods might help Suno AI to improve the quality of its compositions: The system could receive feedback on and learn how to create better over time.
The Role of Music Theory
A significant portion of the capabilities lies in learning patterns from data, however, there is most probably some level of music theory encoded into Suno AI as well. This could include:
- Conventions Surrounding the Harmonic Sequences
- Rhythmic motor learning
- Familiarity with various musical scales and modes
Suno AI is capable of creating sound from these rules and using them allows us to produce compositions that are certain statistically to at least not be bad sounding while adhering at least slightly more towards definite, well-established principles within music.
Challenges and Limitations
As such, while Suno AI can do many impressive things, it faces a number of challenges:
Creativity vs. Imitation: This is an ongoing debate about the merit of AI-generated music, and whether it is actually creative or merely next-level imitation
However, the emotional depth that human composers bring to their work is far less accessible by AI-generated music.
Coherent long-term structure: AI still struggles to produce coherent long-form compositions that sustain through uncommon overarching themes and development.
Copyright and Ethical Issues- Using existing music for training steps into copyright territory, in fact, the whole concept of AI being used to replace a creative industry throws up some troubling circumstances both legally and ethically.
The Future of Suno AI and Music Composition
This advancement is only going to continue as technology gets even more advanced in the years ahead with new editions of Suno AI and its peers. Some potential future ideas are:
- Better Interfaces for Human-AI Collaboration in Musicpaduraru-medium.
- Better songwriting skills, making full songs rather than just beats
- Virtual reality integration allows one to be fully immersed in music
Conclusion
Suno AI is a really interesting fusion of Artificial intelligence and musical creativity. Using high-end machine learning in combination with tons of training data and potentially a little ingrained musical knowledge, it can produce pieces that challenge our perception of creativity within music as well as raise questions about authority.
This will certainly pave the way of new horizons in which both humans and AI can collaborate to explore newer musical sounds. But while it may never attain to the same profundity of human musical creativity, Suno AI and other technologies like it are changing the landscape for music composition and production.
The technology underpinning Suno AI is an example of how quickly and with what force artificial intelligence is transforming a creative field. Welcome to a New Era in Musical Creativity, As we further develop these technologies, the intent is to advance us to an age where human and artificial creation are becoming ever-more fused – opening up new prospects for music in our future.